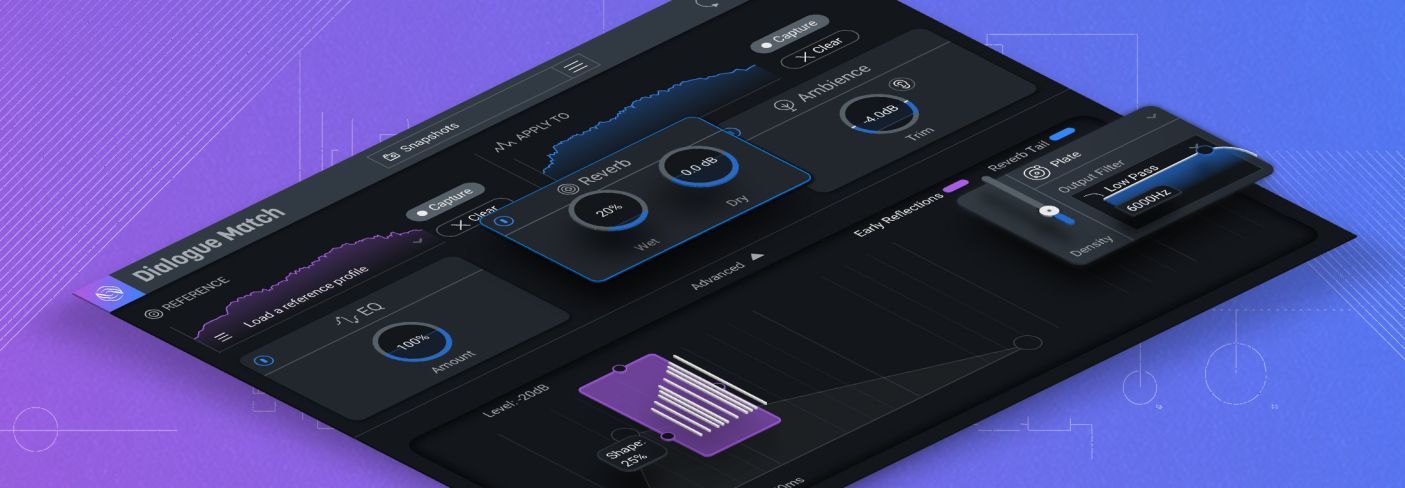
The Technology Behind the Reverb Module in Dialogue Match
Reverb matching is key to Dialogue Match, an iZotope plug-in for dialogue editors and re-recording mixers. Learn more about the development of this revolutionary new tool.
Reverb matching is a key ingredient in Dialogue Match, the newest plug-in from iZotope for dialogue editors and re-recording mixers. Using all-new EQ, reverb, and ambience matching technology, Dialogue Match applies the sonic attributes of one recording directly onto another, giving your scenes environmental and spatial continuity in seconds.
Upon providing a “Reference” and “Apply-To” track, the Reverb module analyzes the reference and produces reverb with similar characteristics, such as reflection density, decay time, color, and size. A simplified view allows you to adjust the wet gain and dry gain, while an advanced panel allows fine-tweaking to your heart's content.
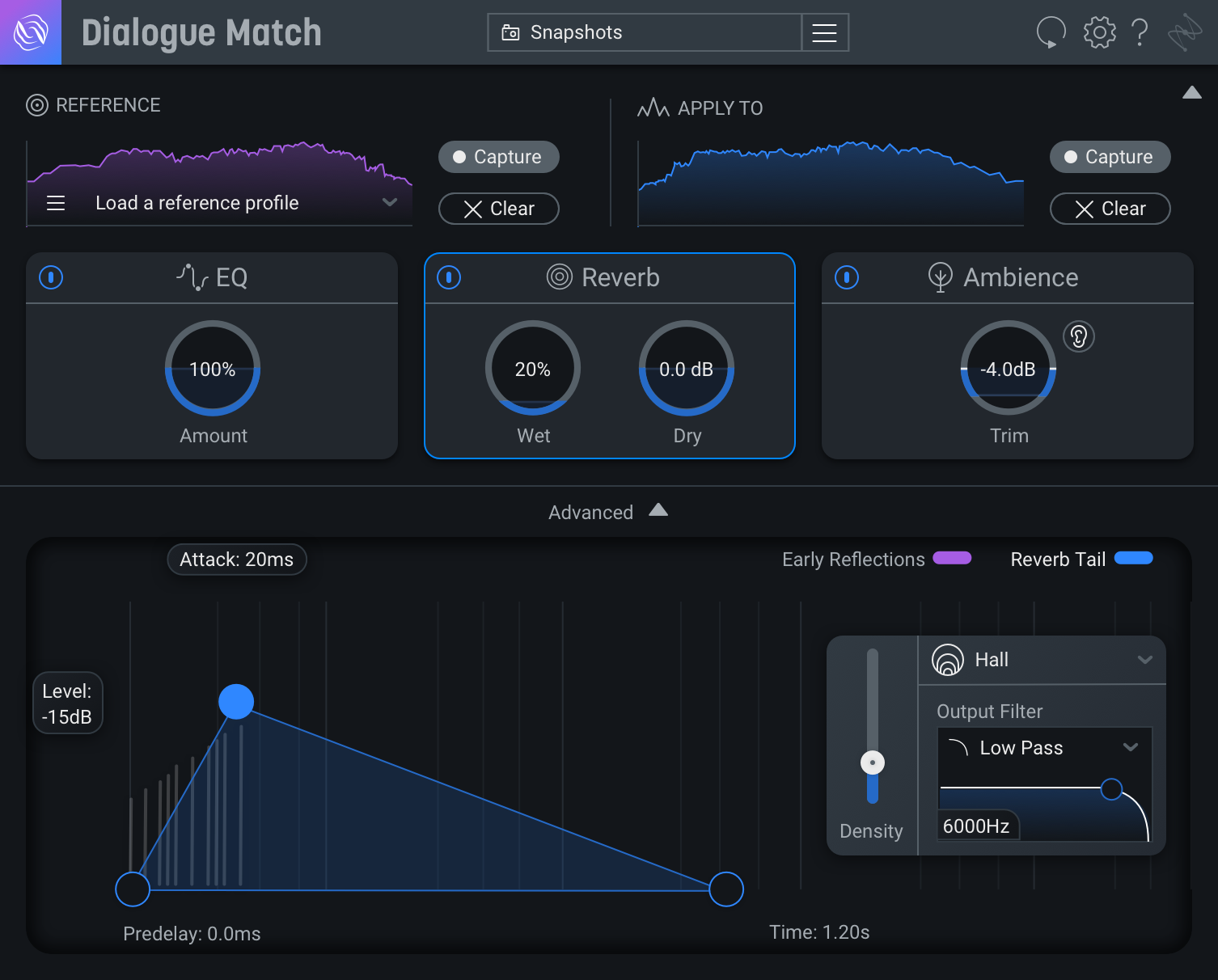
Dialogue Match UI
What is the Reverb module and who is it for?
In dialogue production, we rely on engineers to create consistent, coherent narratives out of audio arriving from multiple sources. For instance, re-recording engineers and mixers need to mix ADR into scenes that already have production dialogue. The ADR must be indistinguishable from originally recorded production dialogue. Documentary editors receive audio and video from several sources and combine them together with voiceover to tell a story. Audio segments might be sourced across multiple years and recorded on different devices and mics, as well as in different environments. Podcasters interview subjects in several spaces, but they want all sources to sound like they’re having a conversation in the same room.
Subtle differences in audio can disorient listeners, distracting them from the narrative. Creating cohesion is integral to providing a good listening experience.
Consistent acoustic cues, such as reverb, are essential to creating the perception that all sources were recorded in the same place. In practice, an engineer might spend hours hand-tweaking artificial reverb parameters to make dry audio sources sound similar to reverberant ones. This is a challenging task because there are nearly infinite combinations of settings. In other words, the engineer must search a parameter space that is incredibly large. On top of this, some combinations of settings interact with each other in nonlinear (read: hard-to-predict) ways.
At iZotope we strive to minimize time spent on tedious tasks so that engineers can focus their skill and creativity where it really counts. Sifting through large, multidimensional, nonlinear parameter spaces takes time and resources most don’t have. Fortunately, machine learning excels at these kinds of tasks. So we asked ourselves, “Is it possible to use machine learning to successfully capture the reverb profile of any naturally reverberated reference track and apply it to a dry track?” It turns out… it is!
Under the hood
The Reverb module employs a neural network to listen to your reverberant reference audio. By providing the network with a large corpus of references, we've trained the network to predict the settings to our reverb DSP. The network analyzes your audio sequentially, using context and examining how it develops over time. Suggestions are provided nearly instantaneously and are influenced by the audio context that is provided.
Behind the scenes, the Reverb module synthesizes reverb using the award-winning technology in Exponential Audio, which has a stellar reputation for providing natural-sounding reverb while offering surgical control over the temporal and tonal characteristics of its reverb. We've trained the neural net to learn an extensive space of parameters driving Exponential Audio’s engine. Given a reference track, the net immediately predicts a unique set of parameters for applying reverb to an unrelated track.
Does it work?
At iZotope, our sound design team is composed of highly experienced listeners who have spent countless hours validating suggestions made by the Reverb module. We also ensured that the reverb matching process surpasses expectations by seeking feedback from external beta testers.
However, we also wanted to check that the assessments of our sound designers and beta testers were supported by cold, hard statistical evidence.
We conducted a MUSHRA experiment to determine whether the Reverb module is as good at matching reverb as an experienced engineer. MUSHRA, which stands for Multiple Stimuli with Hidden Reference and Anchor, is a helpful research tool for understanding how people perceive the similarity of a reference track to a set of comparison tracks. MUSHRA was originally designed to evaluate the quality of audio codecs and has been used in countless research papers on audio similarity and quality.
MUSHRA works like this: there are several trials, and for each trial we provide a clearly labeled reference audio track. In addition, there are several unlabeled and randomly sorted comparison tracks known as the "multiple stimuli." A test volunteer is asked to rate, on a scale of 0–100, the similarity between each stimulus and the reference.
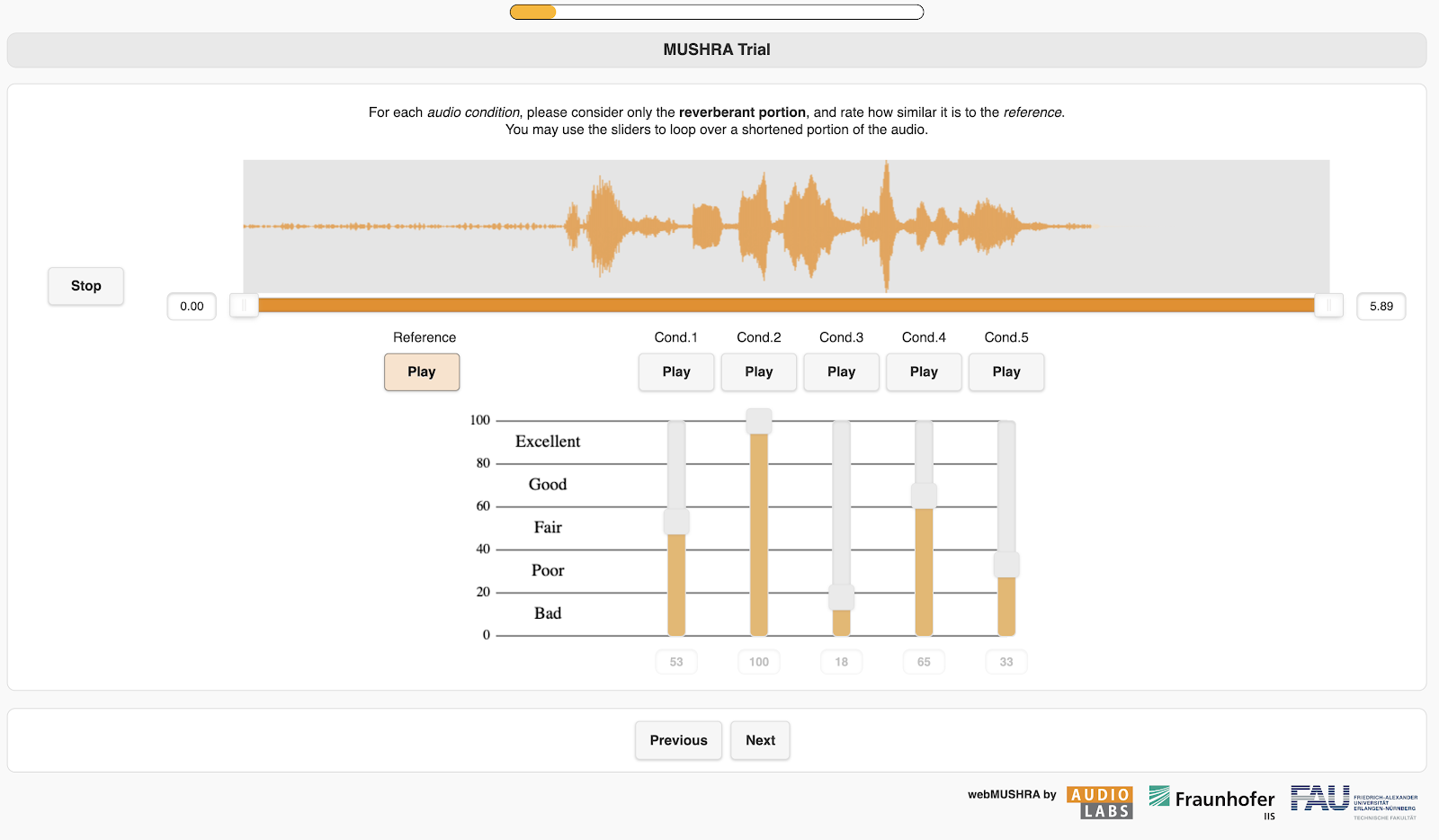
Dialogue Match experiment conducted using webMUSHRA by AudioLabs Schoeffler, M. et al., (2018). webMUSHRA — A Comprehensive Framework for Web-based Listening Tests. Journal of Open Research Software. 6(1), p.8.
One of the stimuli is exactly the same as the reference. This is the "hidden reference." We expect that test volunteers will rate the hidden reference as being very similar to the labeled reference. If not, then we conclude that their ratings are unreliable.
We also insert a very dissimilar anchor in the set of stimuli. It helps us know what the lower range of similarity ratings should be.
Finally, the remaining stimuli are the ones we wish to evaluate. So, in our experiment, we provided the following stimuli:
- The hidden reference
- An anchor, which was a reverberant track made by setting reverb parameters randomly
- Reverb matched by an experienced human engineer
- Reverb matched by two differently-trained neural nets, which we call the “Regression Net” and “Classification Net”
We hoped that we’d find that one of our nets would be rated about as well as our expert engineer. The figure below shows the average ratings of our volunteer testers.
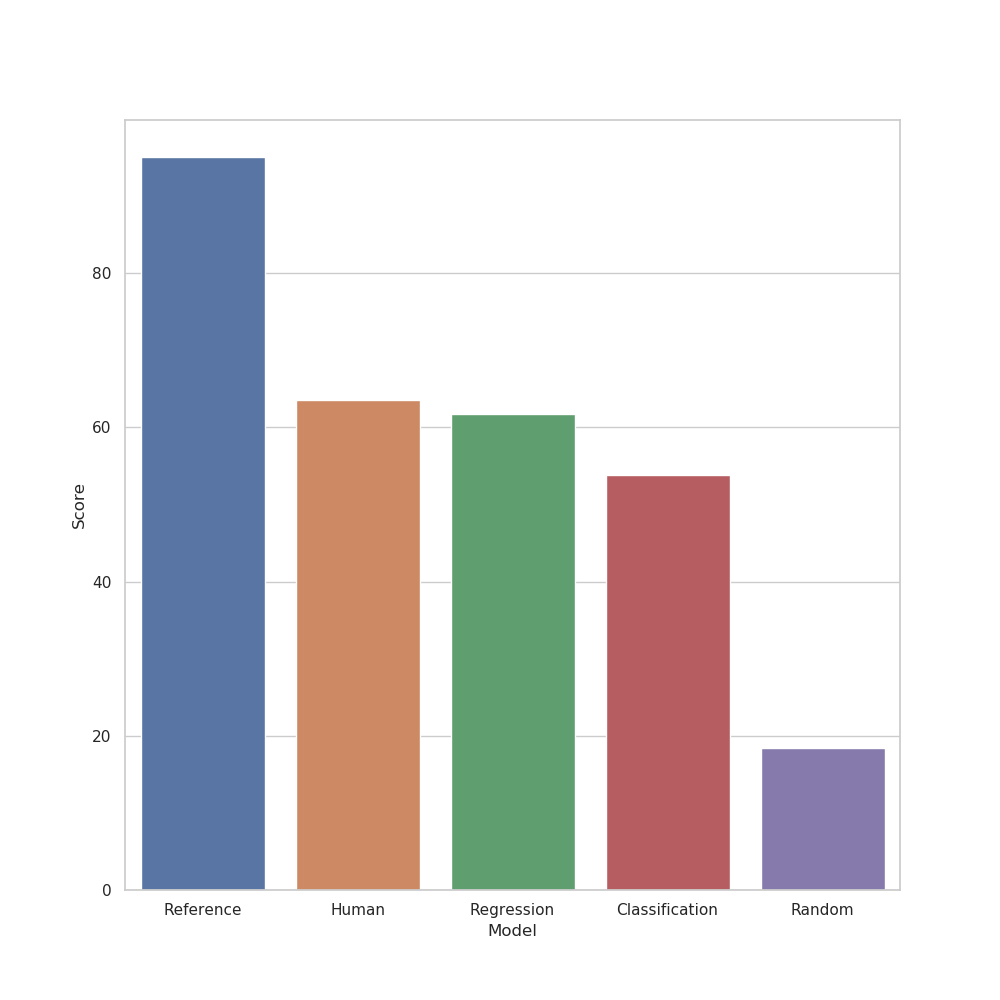
Average rating of volunteer testers
As we mentioned above, we expect the hidden references to receive an average score close to 100 and that randomly reverberated tracks (the anchors) would receive a significantly lower average score. Anything different would suggest a major failure in our experimental design.
We are pleased that the results suggest that the Regression Net (which we built into the Reverb module) is as good as those of the expert engineer. This hypothesis was always supported by our beta testers and sound design team, but now we have some statistics to back us up. More precisely, we can say that, based on a p-value of 0.24, we cannot reject the hypothesis that ratings of a human engineer and our reverb matching are drawn from the same distribution.
Tips to make the Reverb module work best
The neural net behind the Reverb module works best on material with certain characteristics. If you take the following advice you should fare pretty well:
- Provide a reference track of at least three seconds, but not so long that it contains silent or extremely quiet parts. The network was trained on reverberant dialogue of at least three seconds.
- Avoid using noisy reference audio. In particular, the network may be confused by stationary noises such as hum and air conditioner, causing it to overestimate its matches. If your reference is noisy, try denoising it first with tools in RX like De-hum and Spectral De-noise. Learn how to use RX and Dialogue Match together in your workflow here.
- You do not need to provide the full reverb tail of your reference. Doing so might actually hurt performance, as the net may not be so accurate on quiet sections of audio.
- When providing the reference track, be mindful of the length of your Pro Tools clip handles. Reverb Match will analyze the full extent of audio within the handles, including the parts that are not visible. This may lead to some surprising results!
- The Reverb module is intended to be applied to dry audio. If your Apply-To track has some room on it, consider using De-reverb in RX first.
- Finally, keep in mind that the module will adjust its suggestion to different regions of your reference. We recommend trying a few different regions of varying length and saving your reference profiles as you go along.
In conclusion
The Reverb module is a powerful tool inside Dialogue Match that creates acoustic coherence across multiple sources. Although we designed it specifically with dialogue in mind, there's no reason you can't experiment with it on different reference material.
One of the advantages of the feature is that it helps an engineer avoid searching an insurmountably large parameter space, providing a nearly instantaneous prediction, and saving lots of time in the process. In the future, we may consider training neural nets for other large search spaces. So stay tuned for more technology from iZotope.
Be sure to check out Dialogue Match articles and tutorials to learn more about post production workflows, and download your free trial of Dialogue Match.